business and technology ai (artificial intelligence) and machine learning in the cybersecurity battle
The Role of Artificial Intelligence and Machine Learning
Whether we realize it or not, artificial intelligence (AI) and machine learning (ML) play a part in every second of our lives. From the moment we wake, smart devices decide what time to turn on our heaters and our lights, social media uses complex algorithms to select what news to promote to us, and Google Maps navigates us through our day. Even while we sleep, AI monitors our sleeping patterns—with the proliferation of smart devices like Google Home and Apple Watches—identifying when we have had a good night’s sleep and even monitoring our health.
ML and AI in our daily lives have slowly changed how we interact with technology. We use this technology for good by helping the elderly with virtual chatbots, preventing poaching, and providing real-time translation for migrants.
The cybersecurity world has been at the forefront of this technology in the last decade, using artificial intelligence and machine learning in various applications such as tackling huge volumes of malware, detecting spam and business email compromises, analyzing network traffic, using facial recognition, and more. It’s hard to get away from a vendor’s presentation without hearing about their ML and AI nowadays. This blog will demystify and hopefully bring some new angles to our readers in the decision-making around “ML-enabled” security solutions.
What Are Machine Learning and Artificial Intelligence?
Let’s start with simple definitions of machine learning and artificial intelligence. Machine learning involves enabling computers to learn how to do something. This requires input such as training data and knowledge, while AI is the goal of applying the knowledge learned. AI attempts to solve data-based business or technical problems, assisting users in the decision-making process or making judgment itself (if we programmed it in such a way). When it needs to, it can be used to rapidly analyze large sets of data that no human brain could possibly process and can come up with AI-assisted decisions and conclusions on an issue.
Is artificial intelligence perfect? Not always. Any computer program is only as good as its writer, and any ML or AI is only as good as the information it has been fed. There are well-known examples of programmatic biases in some AI algorithms and examples where chatbots have gone rogue after being trained with the wrong data. So, while there is still work to be done, these algorithms can deliver significant benefits over even more fallible humans.
AI-Driven Malware – Myth or Reality?
Despite a large amount of hype and clickbait, there is little evidence to support the belief that criminal cyber gangs are already using AI to help generate new strains of malware, however there is evidence that artificial intelligence and machine learning are being used in other areas to circumvent protective security measures:
Generating deep fake videos and images to phish users and bypass security measures. This is particularly prevalent on social media sites to create fake identities.
Solving CAPTCHAs to bypass authentication protections.
To gather open source intelligence on organizations in order to target attackers.
AI and ML for Cybersecurity: Always Consider Your Use Case
When considering investment priorities among security solutions, evaluate the use cases you’re trying to achieve. Understand how threats are evolving and what tactics and techniques black-hats use. Then ask why you couldn’t stop these attacks with the investment you have so far. It’s pretty easy to get caught up with the AI/ML hype. But customers are starting to move cleverly to consider practical use cases, whether this is detection, forensic, hunting, or mitigation.
How Does Fortinet Use AI and ML-Driven Cybersecurity?
The big change in the malware industry that triggered the need for AI was heuristics and adaptive malware. We went almost overnight from a volume of malware that could be handled manually to a situation with exponential growth in the number of samples. We had to adapt and take advantage of artificial intelligence and machine learning to support our malware analysts.
Fortinet has been in the AI business for more than a decade. At a high level, Fortinet uses artificial intelligence and machine learning in multiple areas:
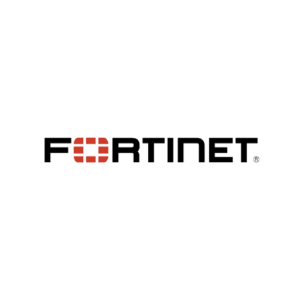
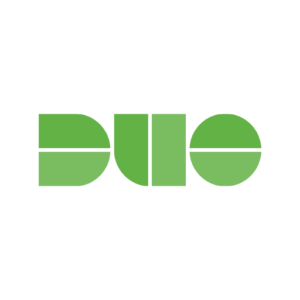

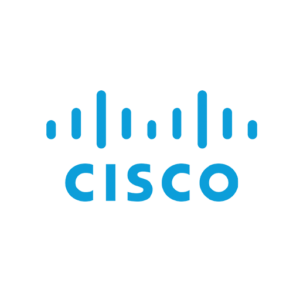
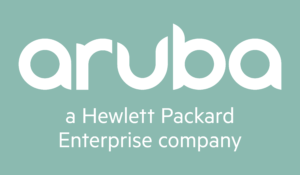

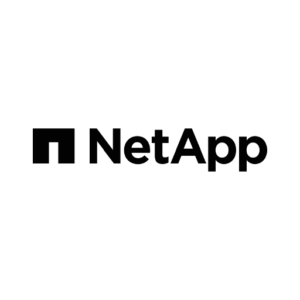
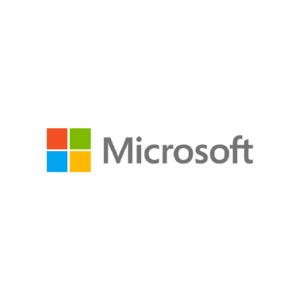
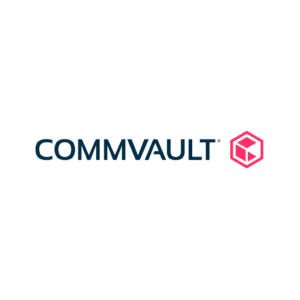
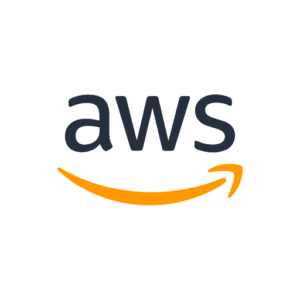
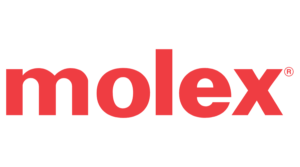
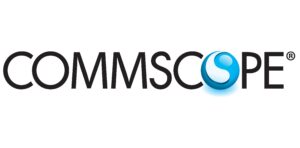
- Scale: One of the first use cases 10 years ago was the advent of the virtual FortiGuard threat analyst. The huge growth in samples meant that analysts could no longer handle the volumes of samples they were receiving, so we created an artificial neural network (ANN) for sub-second sample classification. Over six generations of this solution, this grew into FortiAI, which analyzes millions of samples per day with near-perfect accuracy—a task that would normally require thousands of human analysts.
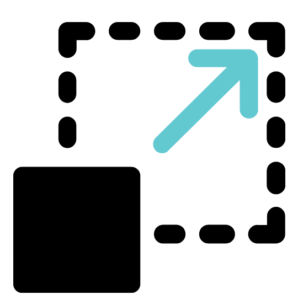
- Enhance: An ML use case is a great way to enhance traditional security solutions. Some examples are:
- Adding ML analysis of malicious vectors in FortiSandbox
- ML-enabled AV engine in FortiOS
- Widely adopt ML in other solutions such as FortiWeb, FortiGuard Security Services, and many more. This enables better and more accurate detections of malicious activities or anomalies for our customers. In this area, innovation is key.

- Predict: ML/AI is particularly good at drawing relationships and making predictions. An example is comparing two infections’ “DNA” and tracing the source of a problem. This is a more advanced application of AI, as prediction has a time element—meaning you can tell ahead of time what will happen. Based on the historical data points, trending, etc., it is possible to predict what might happen to your network.
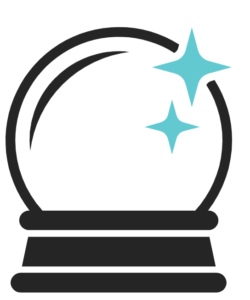
- Reduce time to detect: Fortinet pushes the physical limit to “sub-second” detection of malicious code, enabling SecOps solutions to integrate with our flagship FortiGate NGFW for inline blocking, stopping patient zero. While reducing time to detect from minutes to sub-second might not sound significant, it’s crucial when a major, widespread outbreak occurs. Customers should have the ability to react quickly to threat actors.
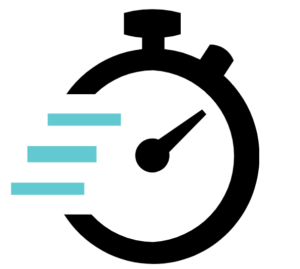
What Makes One ML-Driven Cybersecurity Solution Better Than the Others?
Fortinet embarked on the artificial intelligence and machine learning journey over a decade ago. The Fortinet Security Fabric includes ML and AI applied to the areas that will benefit users the most—whether this is on-premises investigating web traffic, gathering useful NOC data, using face recognition to detect malicious objects, or in the cloud (FortiGuard) for tracking malicious web campaigns, zero-day detection with inference training, and more. Not only are our NGFWs ML-enabled, but we provide a complete ML-enabled Security Fabric. Customers can rely on Fortinet for our experience, innovation, and competitive edge, delivered in our broad, integrated, and automated Security Fabric. In the next decade, watch our space! Fortinet will continue to bring more innovative ML-enabled solutions to our valued customers.
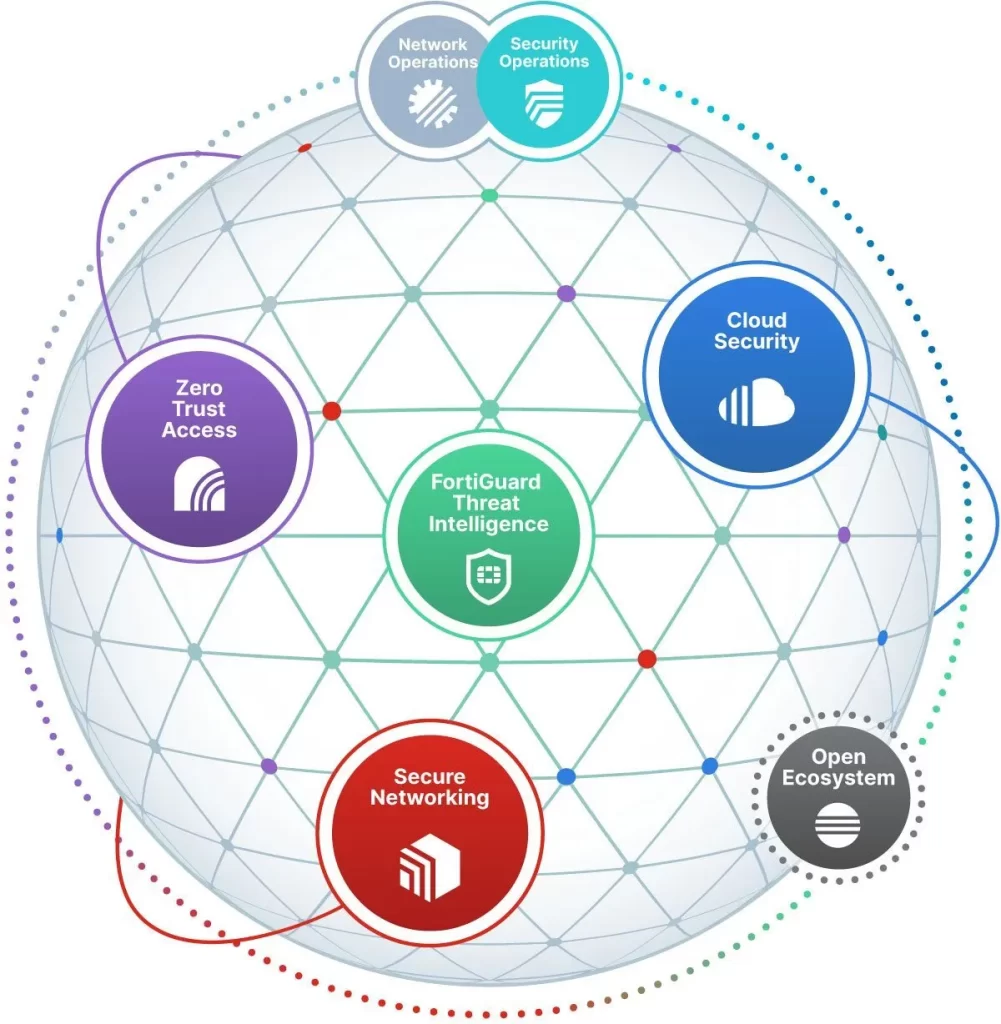